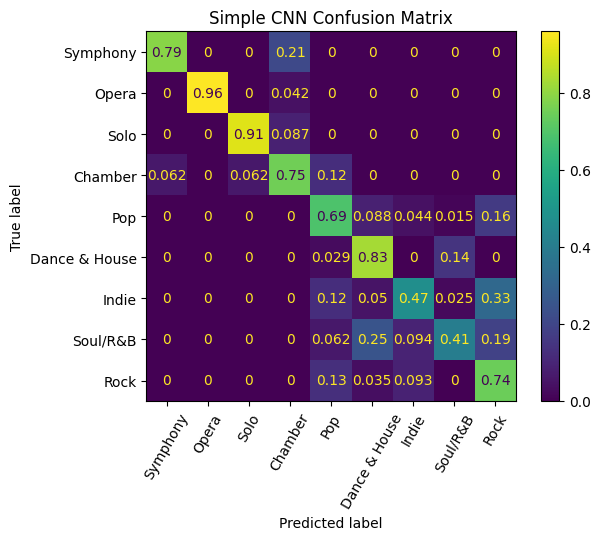
Conclusion
In summary, our exploration into genre classification has yielded commendable results. While our findings may not be considered groundbreaking, the notable achievement lies in the high classification accuracy attained by our CNN model. Additionally, our analysis uncovered discernible patterns in music genre classification, shedding light on statistically significant correlations among certain categories and revealing weaker associations in others.
An essential takeaway from this study is the enhanced understanding of the pivotal role of feature engineering. Notably, the integration of Histogram of Oriented Gradients (HoG) led to substantial improvements across the majority of our models. Looking ahead, we envision the potential for more comprehensive insights by revisiting this project with an expanded dataset and increased processing power. The limitations imposed by the relatively modest dataset of around 1700 spectrograms, coupled with the constrained scope of Western music genres, underscore the need for future iterations of this study to encompass a broader musical landscape.
References
- N. M R and S. Mohan B S, “Music Genre Classification using Spectrograms,” 2020 International Conference on Power, Instrumentation, Control and Computing (PICC), Thrissur, India, 2020, pp. 1-5, doi: 10.1109/PICC51425.2020.9362364.
- Costa, Yandre & Soares de Oliveira, Luiz & Koericb, A.L. & Gouyon, Fabien. (2011). Music genre recognition using spectrograms. Intl. Conf. on Systems, Signal and Image Processing. 1 - 4.
- M. Dong, ‘Convolutional Neural Network Achieves Human-level Accuracy in Music Genre Classification’, CoRR, vol. abs/1802.09697, 2018.
- Zhaorui Liu and Zijin Li, “Music Data Sharing Platform for Computational Musicology Research (CCMUSIC DATASET).” Zenodo, Nov. 12, 2021. doi: 10.5281/ZENODO.5676893.
- M. Hall-Beyer, 2007. GLCM Texture: A Tutorial https://prism.ucalgary.ca/handle/1880/51900 DOI:10.11575/PRISM/33280
- V. Bisot, S. Essid and G. Richard, “HOG and subband power distribution image features for acoustic scene classification,” 2015 23rd European Signal Processing Conference (EUSIPCO), Nice, France, 2015, pp. 719-723, doi: 10.1109/EUSIPCO.2015.7362477.
- Y. Panagakis, C. Kotropoulos and G. R. Arce, “Non-Negative Multilinear Principal Component Analysis of Auditory Temporal Modulations for Music Genre Classification,” in IEEE Transactions on Audio, Speech, and Language Processing, vol. 18, no. 3, pp. 576-588, March 2010, doi: 10.1109/TASL.2009.2036813.
Contributions
Contribution | People Involved |
---|
Neural Implementation | Siddhant |
Analysis | Siddhant |
Visualization, Quantitative Metrics | Soongeol, James |
Presentation | Joseph |
Hyperparameter Tuning | James, Anirudh |
Update Timeline/Contribution Table | Soongeol |
Tuning Code | Everyone |
Results | Everyone |